Artificial intelligence identifies eye disorders and assesses the likelihood of Parkinson's disease by analyzing retinal
images
Chapter 1: Introduction
In recent years, the healthcare industry has seen remarkable advancements in the application of artificial intelligence (AI) to various medical domains. One of the most promising and impactful areas is the analysis of retinal images to identify eye disorders and assess the likelihood of Parkinson's disease. This chapter sets the stage for our exploration by outlining the key motivations, objectives, and significance of using AI in retinal image analysis.
The human eye is a marvel of nature, comprising complex structures like the cornea, lens, iris, and retina. The retina, a thin layer of tissue lining the back of the eye, plays a pivotal role in vision. It consists of specialized cells known as photoreceptors, which capture light and convert it into electrical signals that are transmitted to the brain via the optic nerve. The retina is also home to blood vessels and intricate neural networks, making it an excellent source of information about a person's health.
Chapter 2: The Human Eye and Retinal Imaging
Understanding the human eye's structure and function is fundamental to appreciating the importance of retinal imaging in healthcare. The eye operates like a camera, with the cornea and lens focusing incoming light onto the retina. Retinal imaging involves capturing high-resolution images of this critical structure using specialized cameras and diagnostic tools.
These images reveal various details, including the health of blood vessels, the condition of the optic nerve head, and the presence of abnormalities such as lesions, hemorrhages, or swelling. In addition to diagnosing eye diseases, retinal images can provide valuable insights into systemic conditions like diabetes and hypertension, which often manifest in the eye.
Chapter 3: Eye Disorders and Their Diagnosis
A comprehensive understanding of eye disorders is essential to appreciate the transformative potential of AI in this field. Some of the most common eye conditions include:
Glaucoma: A group of eye diseases that can damage the optic nerve, leading to vision loss.
Diabetic Retinopathy: A complication of diabetes that affects blood vessels in the retina, potentially causing blindness.
Age-Related Macular Degeneration (AMD): A leading cause of vision loss among the elderly, affecting the macula in the central retina.
Retinopathy of Prematurity (ROP): A condition affecting premature infants' retinas, which, if left untreated, can lead to vision impairment.
Ai Productivity Accelerator
Revolutionize your business! Learn exactly how to grow and market your business without spending a bunch of time and money hiring a team.
Read more
Cataracts: Clouding of the eye's lens, causing blurred vision.
Traditional methods of diagnosing these conditions often involve subjective assessments by ophthalmologists and optometrists, which can be time-consuming and prone to human error. AI promises to revolutionize these processes by providing objective, efficient, and accurate diagnostic tools.
Chapter 4: Artificial Intelligence in Healthcare
The introduction of AI in healthcare has ushered in a new era of precision medicine and patient care. AI systems can analyze vast amounts of medical data, including images, patient records, and research articles, with incredible speed and accuracy. This chapter explores the evolution of AI in healthcare, highlighting milestones and breakthroughs that have paved the way for AI-driven retinal image analysis.
Machine learning, a subset of AI, is particularly well-suited for healthcare applications. It encompasses a range of algorithms, with deep learning being the most prominent. Deep learning relies on neural networks, inspired by the human brain, to process and learn from data. These networks can automatically identify patterns and make predictions, making them ideal for tasks like image analysis.
Custom Keto Diet:
Would You Like to Know Exactly What to Eat to Lose Fat and Get Healthy Without Giving Up Your Favorite Foods or Starving Yourself?
I
invite you to read this page
Chapter 5: Deep Learning and Neural Networks
Delving deeper into the technology underpinning AI-driven retinal image analysis, this chapter provides an in-depth look at deep learning and neural networks. At its core, deep learning consists of layers of interconnected nodes, or neurons, that process data. Neural networks can have dozens or even hundreds of layers, allowing them to capture intricate patterns within images and other forms of data.
Convolutional Neural Networks (CNNs) are a specific type of neural network highly effective for image analysis. They use convolutional layers to scan an image for features, gradually building up a hierarchical representation. CNNs have achieved remarkable success in various image-related tasks, from image recognition to medical image analysis.
Chapter 6: The Role of AI in Retinal Image Analysis
With the foundational knowledge of AI and deep learning, this chapter explores how AI is uniquely positioned to transform retinal image analysis. AI algorithms can be trained to recognize subtle abnormalities in retinal images that might elude human observers. They can also analyze large datasets quickly, which is crucial for early diagnosis and treatment.
Moreover, AI systems can provide consistency and objectivity in assessments. The subjectivity that can arise from variations in human interpretation is mitigated, ensuring that patients receive accurate and uniform evaluations.
Scientists Discover A Hidden Root Cause Of Stubborn Belly Fat, And It Will Surprise You…Click
Here to Learn More
Chapter 7: Data Collection and Preprocessing
AI's success in retinal image analysis hinges on high-quality data. This chapter discusses the process of data collection and preprocessing, both of which are critical to training effective AI models.
Data collection involves acquiring a diverse and representative set of retinal images. These images must cover a wide range of conditions, including normal and abnormal cases, to ensure the model's robustness. In addition, metadata, such as patient demographics and clinical history, can be valuable for contextual analysis.
Preprocessing steps are essential to clean and prepare the data for analysis. This may involve resizing images, enhancing contrast, and removing artifacts. Preprocessing helps standardize the input data, making it more suitable for AI algorithms.
Chapter 8: Developing AI Models for Eye Disorders
Building AI models for identifying eye disorders is a complex process that involves multiple steps:
Architecture Selection: Choose an appropriate neural network architecture, often tailored to the specific task, such as detecting diabetic retinopathy.
Data Annotation: Annotate the training dataset by labeling images with the correct diagnosis or relevant features.
Training: Train the model using the annotated dataset, adjusting weights and parameters to minimize errors.
Validation: Assess the model's performance on a separate validation dataset to fine-tune its parameters and prevent overfitting.
Testing and Deployment: Test the model on new, unseen data to evaluate its real-world performance. If successful, deploy the model in clinical settings.
Brand New Probiotics Specially Designed For The Health Of Your Teeth And Gums
Click
Here to Learn More
Chapter 9: Challenges in Retinal Image Analysis
While AI holds great promise, several challenges must be addressed in retinal image analysis:
Data Quality and Quantity: Ensuring that AI models have access to diverse and representative datasets can be challenging due to privacy concerns and data availability.
Interpretability: AI models often operate as "black boxes," making it difficult to understand the rationale behind their predictions.
Regulatory Approval: AI-based medical devices must undergo rigorous testing and receive regulatory approval before widespread adoption.
Human-AI Interaction: Integrating AI into clinical workflows and ensuring effective collaboration between AI systems and healthcare professionals is a complex task.
Ethical Considerations: Balancing the benefits of AI-driven healthcare with ethical concerns, such as data privacy and algorithmic bias, is an ongoing challenge.
Chapter 10: AI and Glaucoma Detection
This chapter focuses on the specific application of AI in glaucoma detection. Glaucoma is a leading cause of irreversible blindness worldwide. AI algorithms can analyze retinal images to detect signs of glaucoma, such as changes in the optic nerve head or thinning of the retinal nerve fiber layer.
Chapter 11: AI and Diabetic Retinopathy
Diabetic retinopathy, a complication of diabetes, can lead to vision loss if not detected and managed early. AI-based systems can screen diabetic patients for retinopathy by analyzing retinal images for characteristic lesions like microaneurysms, hemorrhages, and exudates.
Japanese Biochemist Discovers New 5-second Appetizer That Flushes Out 57 lbs Of Nagging Belly Fat
Click
Here to Learn More
Chapter 12: Age-Related Macular Degeneration
Age-related macular degeneration (AMD) primarily affects the macula, a region in the central retina responsible for sharp central vision. AI can assist in diagnosing AMD by identifying drusen deposits, pigmentary changes, and other characteristic features in retinal images.
Chapter 13: Retinopathy of Prematurity (ROP)
ROP is a condition affecting premature infants, and early diagnosis and treatment are crucial to preventing vision impairment. AI can aid in the screening of ROP by analyzing retinal images to detect abnormal blood vessel development.
Chapter 14: AI in Parkinson's Disease Assessment
Shifting our focus from eye disorders, this chapter explores how AI can assess the likelihood of Parkinson's disease through retinal imaging. Parkinson's disease is a neurodegenerative disorder characterized by motor and non-motor symptoms, and its early diagnosis is challenging. However, recent research suggests that retinal changes could serve as biomarkers for Parkinson's disease.
Chapter 15: Understanding Parkinson's Disease
To understand how AI can assess the likelihood of Parkinson's disease through retinal imaging, it's crucial to have a comprehensive understanding of the condition itself. Parkinson's disease is a progressive neurological disorder that affects movement control. Its hallmark features include tremors, bradykinesia (slowness of movement), rigidity, and postural instability.
Are you looking for the hottest blood sugar support and type 2 diabetes in the market right now? Look no further!
Click
Here to Discover More
Chapter 16: Linking Parkinson's Disease to the Retina
Recent studies have explored the link between Parkinson's disease and the retina. Researchers have observed that changes in the retina, such as thinning of the retinal nerve fiber layer and altered blood vessel morphology, may correlate with the presence and progression of Parkinson's disease. AI plays a pivotal role in identifying and quantifying these retinal changes.
Chapter 17: The Quest for Biomarkers
Biomarkers are measurable indicators of biological processes or disease states. In the context of Parkinson's disease, finding reliable biomarkers is crucial for early diagnosis and monitoring. AI-driven analysis of retinal images offers a non-invasive and accessible approach to identifying potential biomarkers associated with the disease.
Chapter 18: Developing AI Models for Parkinson's Assessment
The development of AI models for assessing the likelihood of Parkinson's disease through retinal imaging involves unique challenges and considerations. Researchers must:
Curate Datasets: Gather diverse datasets that include retinal images from individuals with and without Parkinson's disease.
Feature Extraction: Extract relevant features from retinal images, such as vessel parameters, thickness of retinal layers, and other biomarkers.
Model Training: Train AI models, often leveraging deep learning architectures, to learn patterns and associations between retinal features and Parkinson's disease.
Validation and Testing: Rigorously validate models on independent datasets to ensure their accuracy and generalizability.
Clinical Integration: Work towards the integration of AI models into clinical practice, where they can assist healthcare professionals in identifying at-risk individuals.
Weight
Loss ... The most talked about weight loss product is finally here! BioFit is a powerful supplement that supports healthy weight loss the natural way.
Read
more
Chapter 19: Clinical Trials and Validation
AI models for assessing Parkinson's disease risk based on retinal images must undergo rigorous validation through clinical trials. This
addresses the process of validation, including the importance of large and diverse patient cohorts, validation metrics, and regulatory approval considerations.
Chapter 20: Real-world Applications
Real-world applications demonstrate the practical impact of AI in healthcare. This
showcases successful case studies and real-world scenarios where AI-driven retinal image analysis has made a difference. These examples range from large-scale screening programs to personalized medicine approaches.
Chapter 21: Ethical and Privacy Considerations
The integration of AI in healthcare raises ethical and privacy concerns. Patient data privacy, algorithmic transparency, and bias mitigation are paramount. This chapter delves into the ethical considerations associated with AI in retinal image analysis and highlights the importance of patient consent and data security.
The Plant-Based Recipe Cookbook
- "Want To Cook Ridiculously Tasty Vegan Recipes From Scratch But Have No Idea Where To Start?"
Read
more
Chapter 22: The Future of AI in Retinal Image Analysis
The future of AI in retinal image analysis holds great promise. This chapter speculates on potential advancements, including:
Increased Accuracy: AI models will become even more accurate in detecting eye disorders and assessing disease risk.
Personalized Medicine: AI will enable personalized treatment plans based on a patient's retinal characteristics and medical history.
Telemedicine: Remote retinal image analysis will become more accessible, improving healthcare access for underserved populations.
Multimodal Analysis: AI will integrate data from various sources, such as genetic information and electronic health records, for a comprehensive patient profile.
AI in Drug Discovery: AI-driven analysis of retinal images may contribute to drug discovery efforts for neurodegenerative diseases like Parkinson's.
Chapter 23: Challenges and Limitations of AI in Healthcare
Despite its potential, AI in healthcare faces several challenges:
Data Quality: AI models require large, high-quality datasets, which can be challenging to obtain.
Interoperability: Integrating AI into existing healthcare systems can be complex due to compatibility issues.
Regulatory Hurdles: Navigating regulatory frameworks, such as FDA approval, can be time-consuming and costly.
Bias and Fairness: Ensuring AI systems are unbiased and fair, especially in healthcare, is a critical concern.
Ethical Dilemmas: Balancing the benefits of AI with ethical considerations, such as patient privacy, is an ongoing challenge.
Chapter 24: The Human-AI Partnership
Effective collaboration between AI systems and healthcare professionals is essential. AI can assist clinicians by providing data-driven insights, but human expertise remains irreplaceable. This
explores how the human-AI partnership can optimize patient care, enhance diagnostics, and improve treatment outcomes.
Chapter 25: Patient Perspectives
To gain a holistic understanding of AI-driven retinal image analysis, this chapter features patient perspectives. We hear from individuals who have undergone retinal imaging and experienced the impact of AI in their healthcare journey. Their stories provide valuable insights into the patient experience and the potential of AI to transform lives.
Chapter 26: Conclusion
In this final chapter, we recap the key takeaways from our comprehensive exploration of AI's role in identifying eye disorders and assessing the likelihood of Parkinson's disease through retinal image analysis. We emphasize the transformative potential of AI in revolutionizing healthcare, improving early disease detection, and ultimately enhancing patient outcomes. We conclude by looking ahead to the promising future of AI in healthcare and the continued advancement of this field.
Featured books
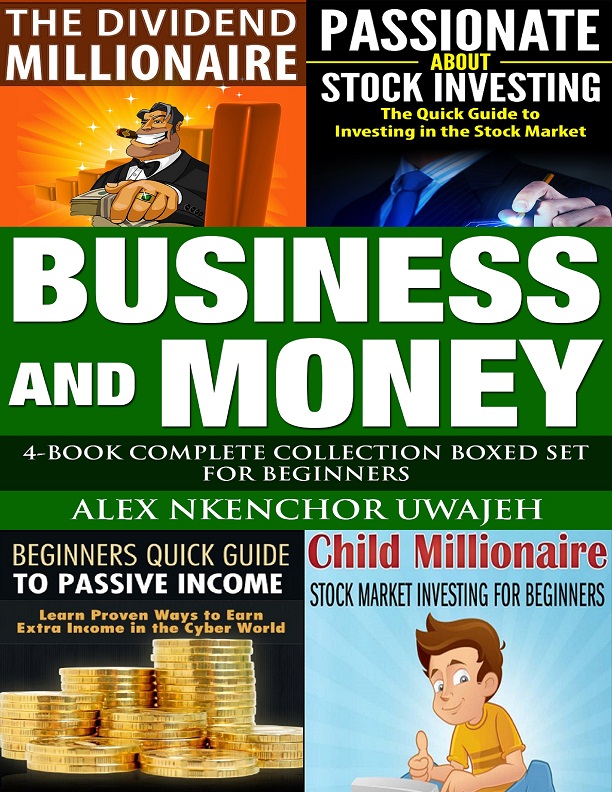
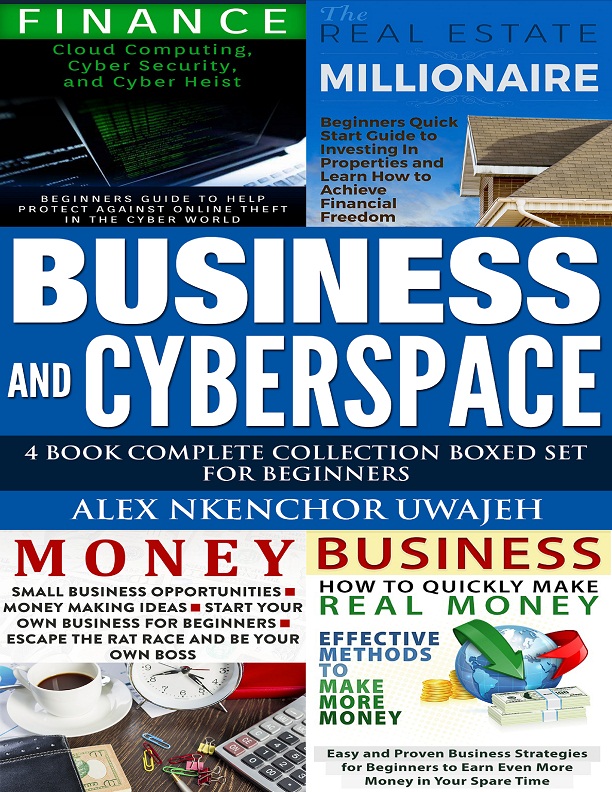
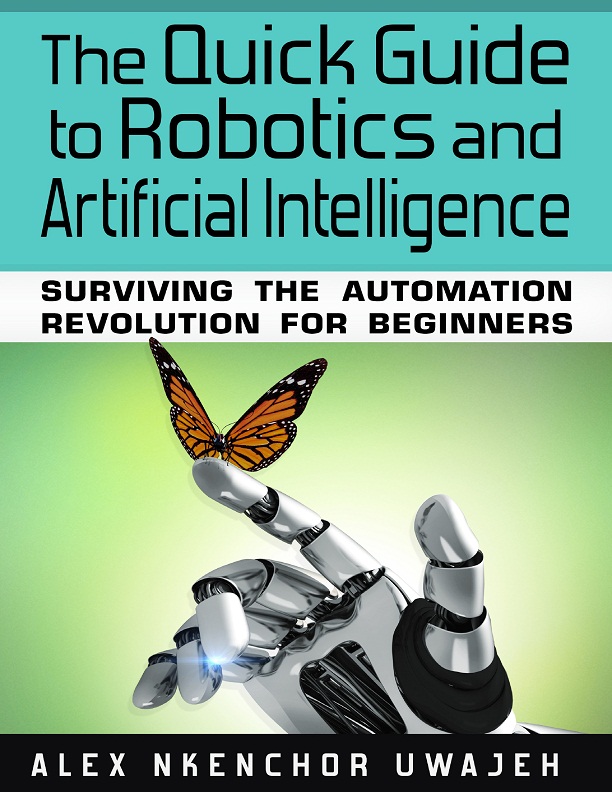
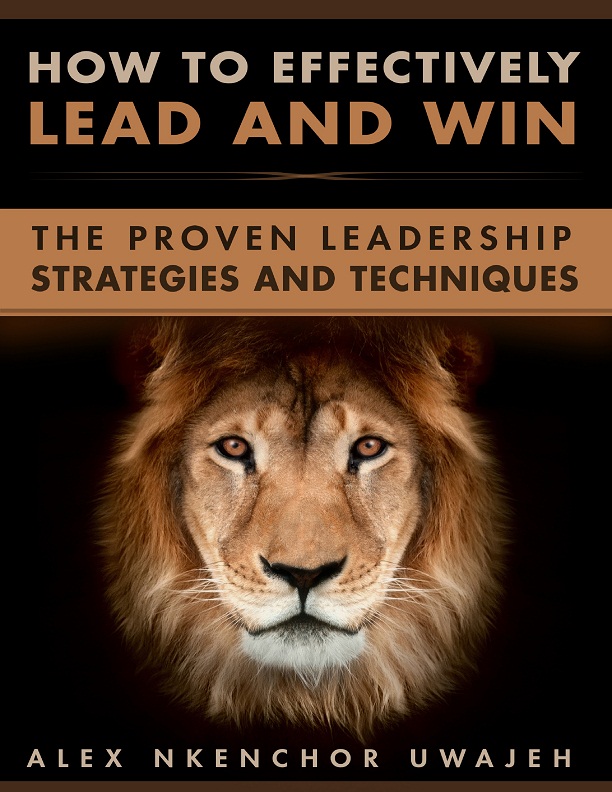
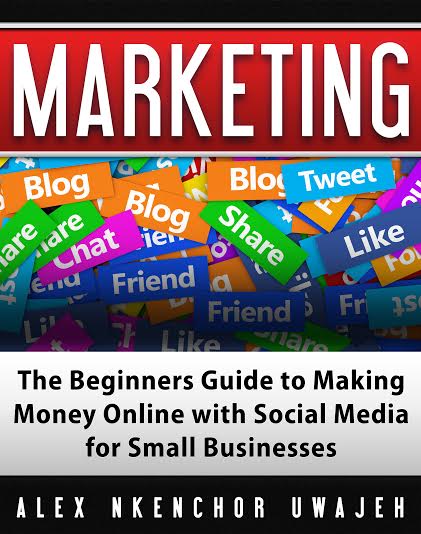
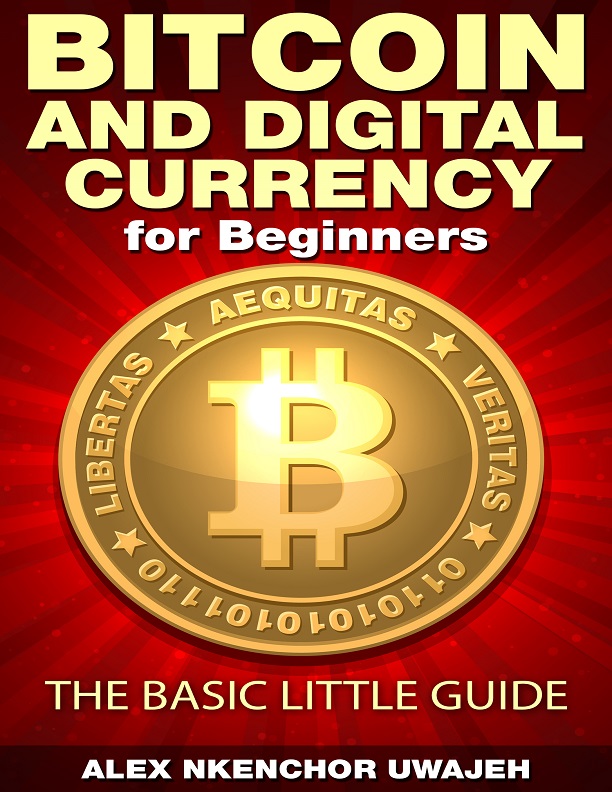
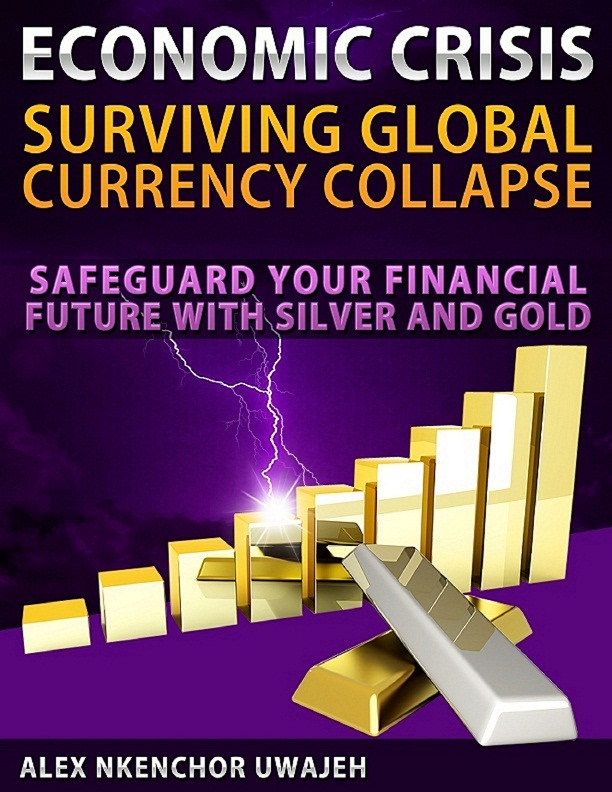
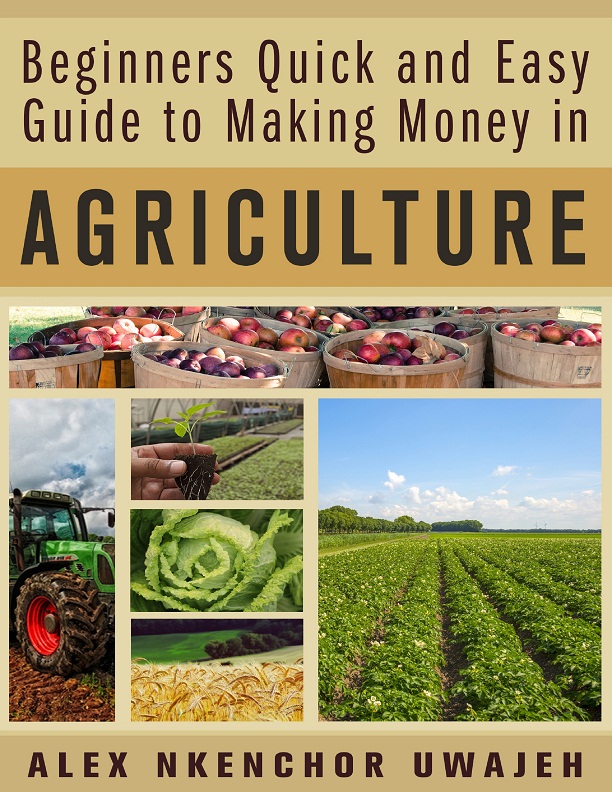
Browse
my Google Playstore Books
Buy
at Amazon
Want
Audible Audio Books? Start Listening Now, 30 Days Free
Return
to Home Page
|