Unmasking AI Bias: Uncovering Hidden Prejudices in Machine Learning Algorithms
Introduction to AI Bias
In recent years, Artificial Intelligence (AI) has revolutionized various industries, ranging from healthcare and finance to education and transportation. Machine learning algorithms, a subset of AI, have the ability to analyze vast amounts of data and make predictions or decisions based on patterns they identify. However, these algorithms are not infallible and can inherit biases present in the data they are trained on or the way they are designed.
Ai Productivity Accelerator
Revolutionize your business! Learn exactly how to grow and market your business without spending a bunch of time and money hiring a team.
Read more
AI bias refers to the systematic and unfair favoritism or discrimination towards certain groups, individuals, or outcomes within AI systems. It can stem from historical societal biases or be introduced inadvertently during the development and training process. While AI bias can sometimes be subtle, its effects can have significant consequences in various domains, perpetuating social inequalities and marginalizing certain populations.
Addressing AI bias is crucial for several reasons. First, biased AI algorithms can reinforce and exacerbate existing prejudices, further entrenching societal disparities. Second, biased AI systems can lead to unfair treatment of individuals, impacting their opportunities, rights, and well-being. Third, it can undermine public trust in AI technologies, hindering their broader adoption and potential benefits.
Custom Keto Diet:
Would You Like to Know Exactly What to Eat to Lose Fat and Get Healthy Without Giving Up Your Favorite Foods or Starving Yourself?
I
invite you to read this page
Understanding Machine Learning Algorithms
To comprehend AI bias, it's essential to grasp the fundamentals of machine learning algorithms. Machine learning involves training a model on a dataset to learn patterns and make predictions or decisions on new data. Supervised learning relies on labeled data to learn from input-output pairs, while unsupervised learning finds patterns in unlabeled data. Reinforcement learning involves agents interacting with an environment and learning from rewards or penalties.
Types of AI Bias
Various types of AI bias can arise in different stages of AI development and deployment:
Explicit Bias: Bias explicitly programmed into the algorithms, resulting from human decisions.
Implicit Bias: Bias indirectly learned from the data and patterns it contains.
Systemic Bias: Bias originating from systemic societal inequalities reflected in the data.
Data Bias: Bias present in the training data due to historical or social factors.
Algorithmic Bias: Bias emerging from the algorithm's design and decision-making process.
Brand New Probiotics
Specially Designed For The
Health Of Your Teeth And Gums
(Hint - No Toothpaste or Mouthwash Involved)...Click
Here to Learn More
Historical Cases of AI Bias
Examining past incidents of AI bias is crucial to understand the real-world impact of biased AI systems. Instances like biased facial recognition algorithms misidentifying people of color or gender-based discrimination in AI hiring tools serve as cautionary tales highlighting the urgent need to tackle AI bias.
The Role of Data in AI Bias
Data is the foundation of AI models, and its quality and representativeness are critical. Biased data, either due to underrepresentation, overrepresentation, or labeling errors, can propagate biases in AI outputs. Data collection methods, biases in data sources, and biased data sampling should be carefully considered to mitigate AI bias.
Scientists Discover A Hidden Root Cause Of Stubborn Belly Fat, And It Will Surprise You…Click
Here to Learn More
Uncovering Algorithmic Bias
Algorithmic bias can occur in various ways. Sometimes, it arises from biased training data, while in other cases, it results from the structure and complexity of the algorithms themselves. Techniques such as sensitivity analysis and causal modeling can help identify and understand algorithmic biases.
Bias in Natural Language Processing (NLP)
NLP algorithms often reflect societal biases present in the language data they learn from. This can lead to biased language models, gendered language, or offensive outputs. Techniques like debiasing and adversarial training can help address NLP bias.
Bias in Image Recognition
AI systems that analyze images can inadvertently learn biased associations between certain attributes and specific groups. Understanding the sources of bias in image recognition models and using diverse and representative image datasets are essential steps in reducing image recognition bias.
Bias in Facial Recognition Technology
Facial recognition technology has raised significant concerns about privacy and bias. Biases in facial recognition algorithms can result in misidentifications, disproportionately affecting certain ethnicities or genders. Transparent evaluation methods and regulations are vital to ensuring the ethical use of facial recognition technology.
Brand New Probiotics Specially Designed For The Health Of Your Teeth And Gums
Click
Here to Learn More
Bias in Healthcare AI
Healthcare AI systems have the potential to improve patient outcomes, but they must be free from bias to ensure fair and accurate treatment. Addressing healthcare disparities, considering sensitive patient data, and regular audits of AI models can help reduce bias in healthcare AI.
Bias in AI Hiring Tools
AI-powered hiring tools can inadvertently discriminate against certain groups, leading to biased hiring decisions. Fairness-aware AI algorithms and continuous monitoring of their performance can enhance the fairness and transparency of AI hiring tools.
Japanese Biochemist Discovers New 5-second Appetizer That Flushes Out 57 lbs Of Nagging Belly Fat
Click
Here to Learn More
Socio-economic Implications of AI Bias
AI bias can perpetuate societal inequalities and disproportionately affect marginalized communities. Recognizing these socio-economic implications is vital for creating fair AI systems and ensuring that technology benefits all members of society.
Legal and Ethical Aspects of AI Bias
Several legal and ethical frameworks govern AI development and deployment, but their effectiveness in addressing bias varies. Striking a balance between innovation and regulation is crucial to mitigate AI bias effectively.
Mitigating AI Bias: Pre-processing Techniques
Pre-processing techniques aim to reduce bias in the data before training the AI model. Techniques such as data re-sampling, data augmentation, and removing sensitive attributes can help mitigate data bias.
Are you looking for the
hottest blood sugar support and type 2 diabetes in the market right now? Look no further!
Click
Here to Discover More
Mitigating AI Bias: In-processing Techniques
In-processing techniques focus on reducing bias during the model training process. Algorithms like adversarial learning and equalized odds optimization can enhance fairness in AI decision-making.
Mitigating AI Bias: Post-processing Techniques
Post-processing techniques involve adjusting AI model outputs to ensure fairness. Techniques like re-calibration and post-hoc fairness can help align AI decision-making with ethical standards.
Building Diverse and Inclusive AI Teams
Diverse and inclusive AI development teams can bring different perspectives and experiences to the table, reducing the likelihood of biased outcomes. Encouraging diversity in the AI field is crucial for fostering ethical AI development.
Weight
Loss ... The most talked about weight loss product is finally here! BioFit is a powerful supplement that supports healthy weight loss the natural way.
Read
more
Collaborative Efforts in Bias Mitigation
Addressing AI bias requires collaboration between academia, industry, policymakers, and advocacy groups. Joint efforts can lead to better policies, guidelines, and tools for identifying and mitigating AI bias.
AI Bias Auditing
AI bias auditing involves systematic evaluations of AI systems for bias detection and mitigation. Independent auditors and standardized evaluation protocols can improve transparency and accountability in AI development.
User Awareness and Education
Educating users about AI bias and its implications can foster responsible AI usage. Empowering users to question AI outputs and understand the limitations of AI models is crucial for promoting fairness.
The Future of AI Bias
The future of AI bias mitigation involves ongoing research, innovation, and collaboration. Integrating fairness into AI development pipelines and adopting ethical AI certification standards can pave the way for a bias-free future.
Case Studies in Bias Mitigation
Case studies showcasing successful bias mitigation strategies can inspire others to adopt similar approaches. Learning from real-world examples can provide valuable insights for tackling AI bias effectively.
Ethical AI Certification
Establishing standardized ethical AI certification frameworks can help ensure the development and deployment of fair and unbiased AI systems. Certification can signal trustworthiness and accountability in AI applications.
The Plant-Based Recipe Cookbook
- "Want To Cook Ridiculously Tasty Vegan Recipes From Scratch But Have No Idea Where To Start?"
Read
more
Advocating for Bias-Free AI
Advocacy efforts can create awareness about AI bias and drive policy changes. Collaborating with policymakers, AI developers, and stakeholders can lead to meaningful progress in addressing AI bias.
Conclusion
In conclusion, unmasking AI bias is crucial to building a fair and inclusive future. By understanding the various sources and types of AI bias and implementing comprehensive mitigation strategies, we can ensure that AI technologies benefit society as a whole, free from hidden prejudices. Ethical AI development, user education, interdisciplinary collaboration, and continuous auditing are vital steps towards achieving unbiased AI systems and harnessing the true potential of artificial intelligence.
Featured books
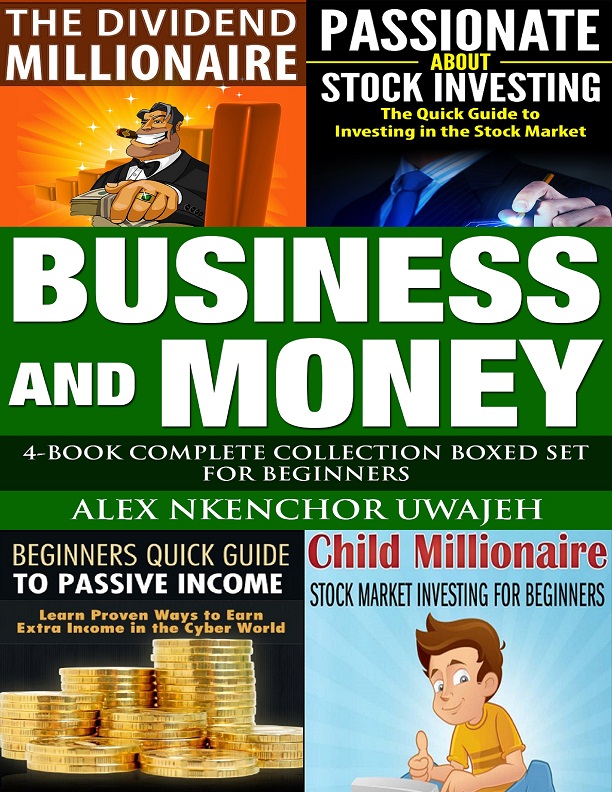
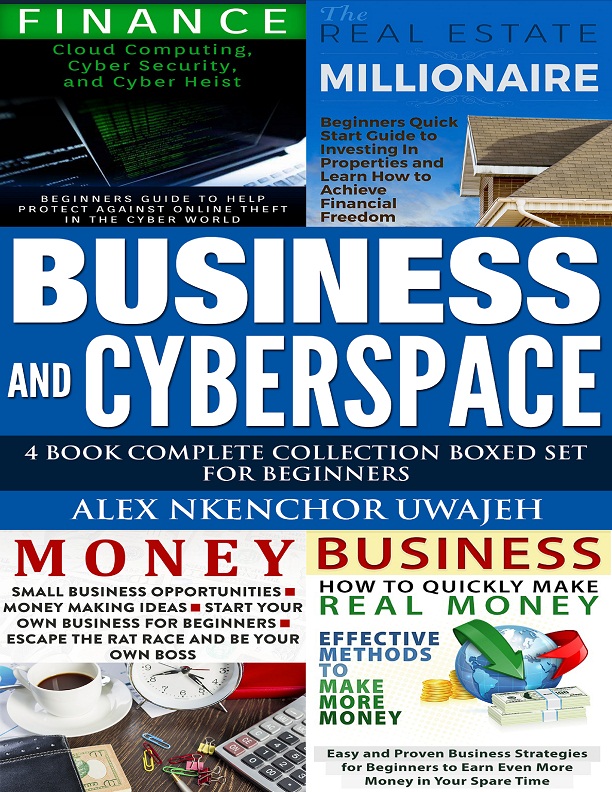
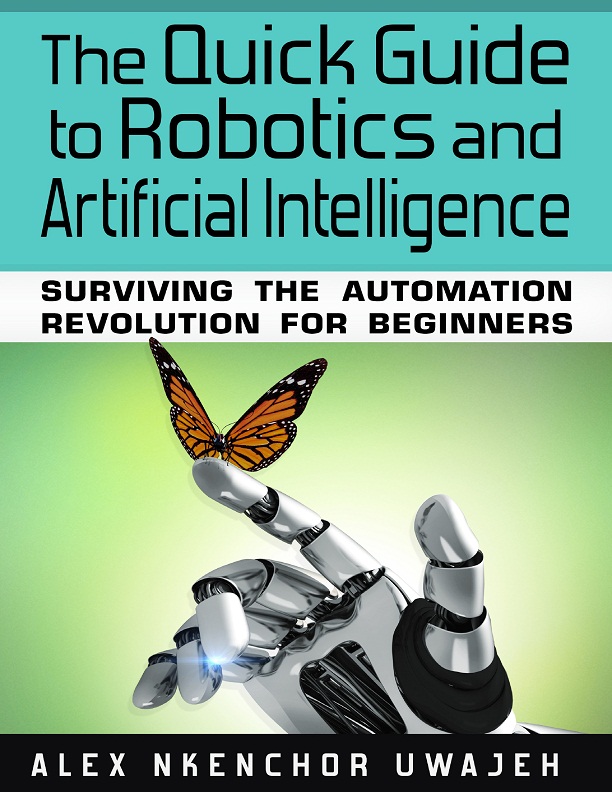
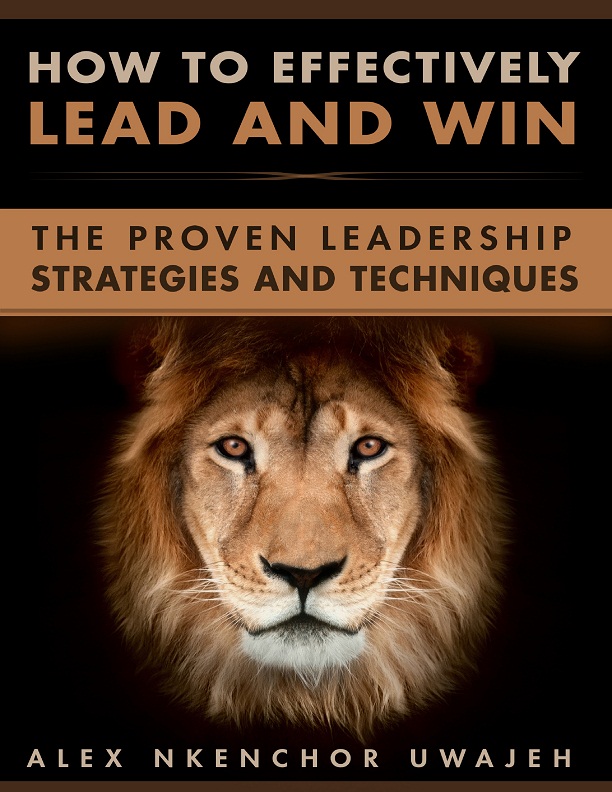
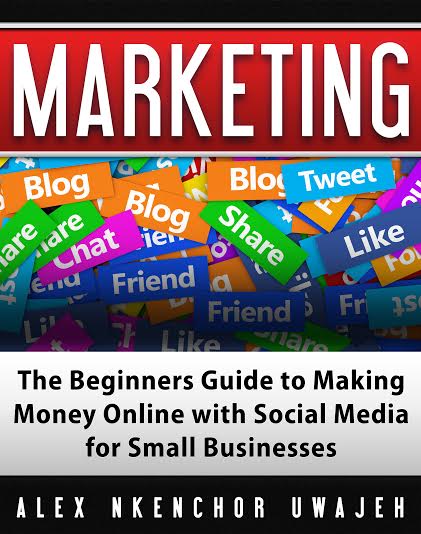
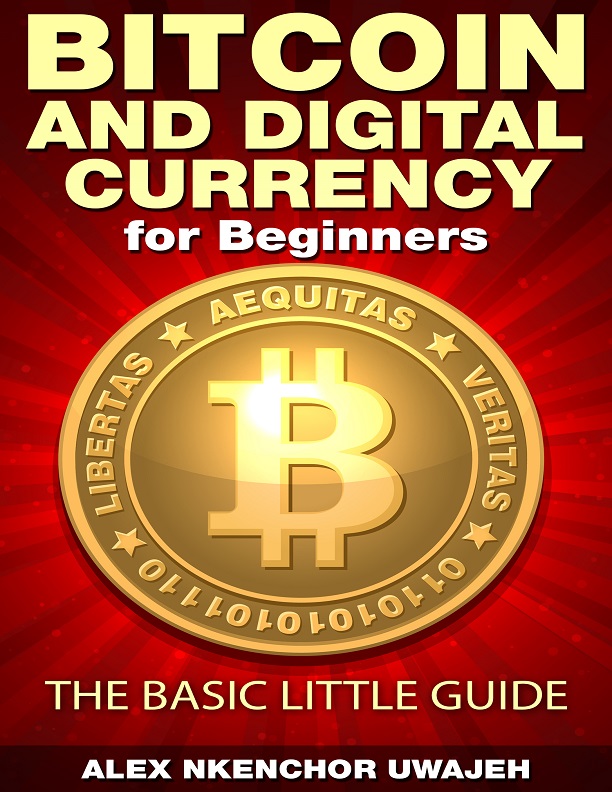
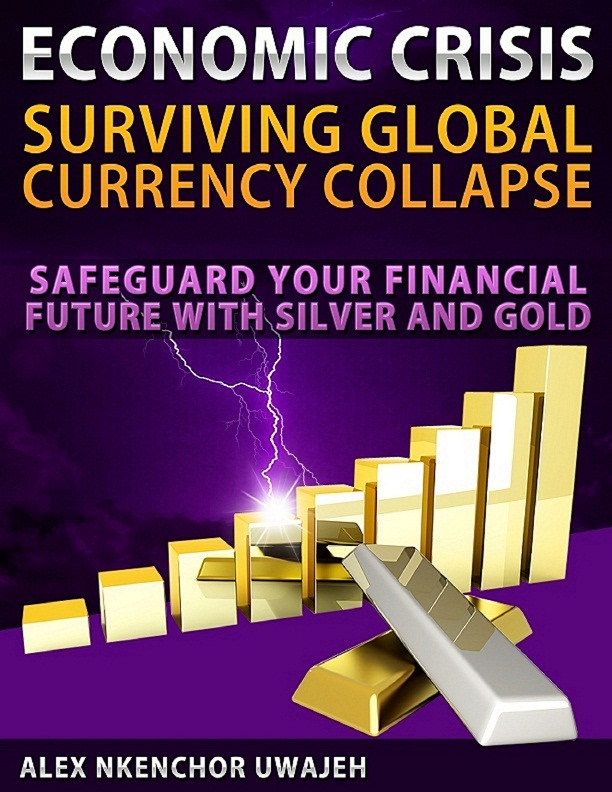
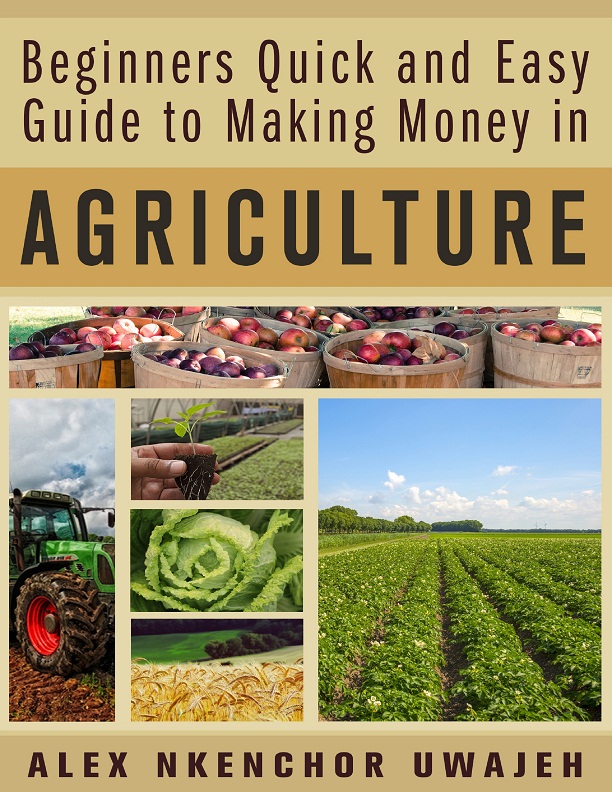
Browse
my Google Playstore Books
Buy
at Amazon
Want
Audible Audio Books? Start Listening Now, 30 Days Free
Return
to Home Page
|